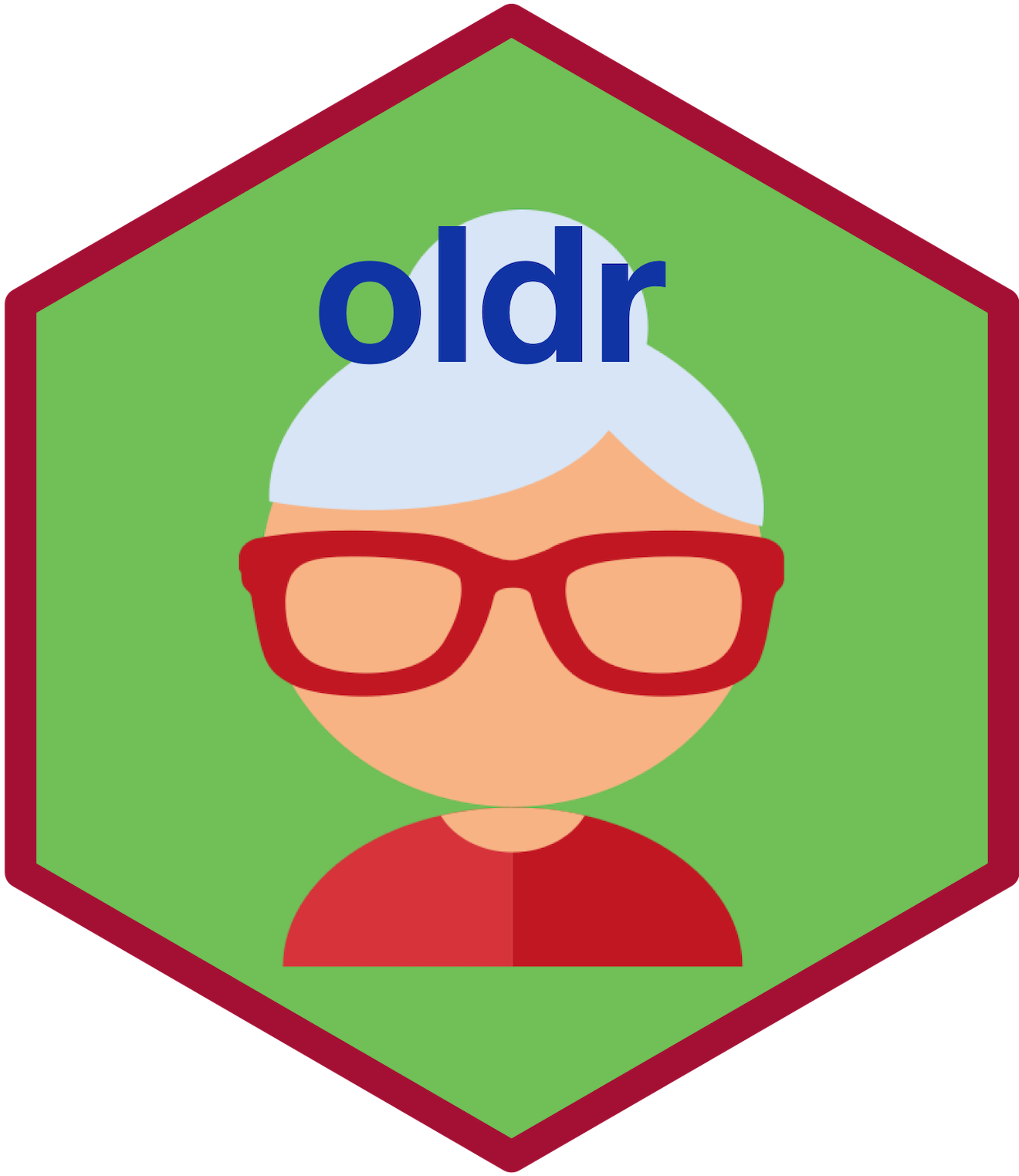
Function to apply bootstrap to RAM-OP indicators using a classical estimator.
Source:R/05-classicBoot.R
estimate_classic.Rd
Function to apply bootstrap to RAM-OP indicators using a classical estimator.
Usage
estimate_classic(
x,
w,
statistic = bbw::bootClassic,
indicators = c("demo", "food", "hunger", "adl", "disability", "mental", "dementia",
"health", "oedema", "screening", "income", "wash", "visual", "misc"),
params = get_variables(indicators),
outputColumns = params,
replicates = 399
)
Arguments
- x
Indicators dataset produced by create_op_all with primary sampling unit (PSU) in column named
PSU
- w
A data frame with primary sampling unit (PSU) in column named
psu
and survey weight (i.e. PSU population) in column namedpop
- statistic
A function operating on data in
x
; fixed to bootClassic function for means- indicators
A character vector of indicator set names to estimate. Indicator set names are
demo
,food
,hunger
,disability
,adl
,mental
,dementia
,health
,income
,wash
,visual
, andmisc
. Default is all indicator sets.- params
Parameters (named columns in
x
) passed to the function specified instatistic
. This is equivalent to variables corresponding to the indicator sets specified inindicators
. The function get_variables is used to specify these variables.- outputColumns
Names of columns in output data frame. This defaults to values specified in
params
- replicates
Number of bootstrap replicates
Examples
#
test <- estimate_classic(x = indicators.ALL,
w = testPSU,
replicates = 9)
test
#> # A tibble: 136 × 10
#> INDICATOR EST.ALL LCL.ALL UCL.ALL EST.MALES LCL.MALES UCL.MALES EST.FEMALES
#> <chr> <dbl> <dbl> <dbl> <dbl> <dbl> <dbl> <dbl>
#> 1 resp1 0.859 0.794 0.880 0.803 0.743 0.835 0.849
#> 2 resp2 0.0885 0.075 0.152 0.1 0.0308 0.125 0.123
#> 3 resp3 0.0417 0.0219 0.0729 0.0658 0.0309 0.166 0.0169
#> 4 resp4 0 0 0.0229 0.0132 0 0.0889 0.00826
#> 5 age 70.7 69.4 72.1 71.0 69.3 72.5 71.1
#> 6 ageGrp1 0 0 0 0 0 0 0
#> 7 ageGrp2 0.536 0.441 0.586 0.470 0.423 0.609 0.517
#> 8 ageGrp3 0.229 0.181 0.328 0.325 0.211 0.380 0.218
#> 9 ageGrp4 0.198 0.123 0.277 0.153 0.0890 0.268 0.272
#> 10 ageGrp5 0.0365 0.0219 0.0573 0.0488 0.0236 0.0747 0.0391
#> # ℹ 126 more rows
#> # ℹ 2 more variables: LCL.FEMALES <dbl>, UCL.FEMALES <dbl>